presenting the amazing evolving automatons
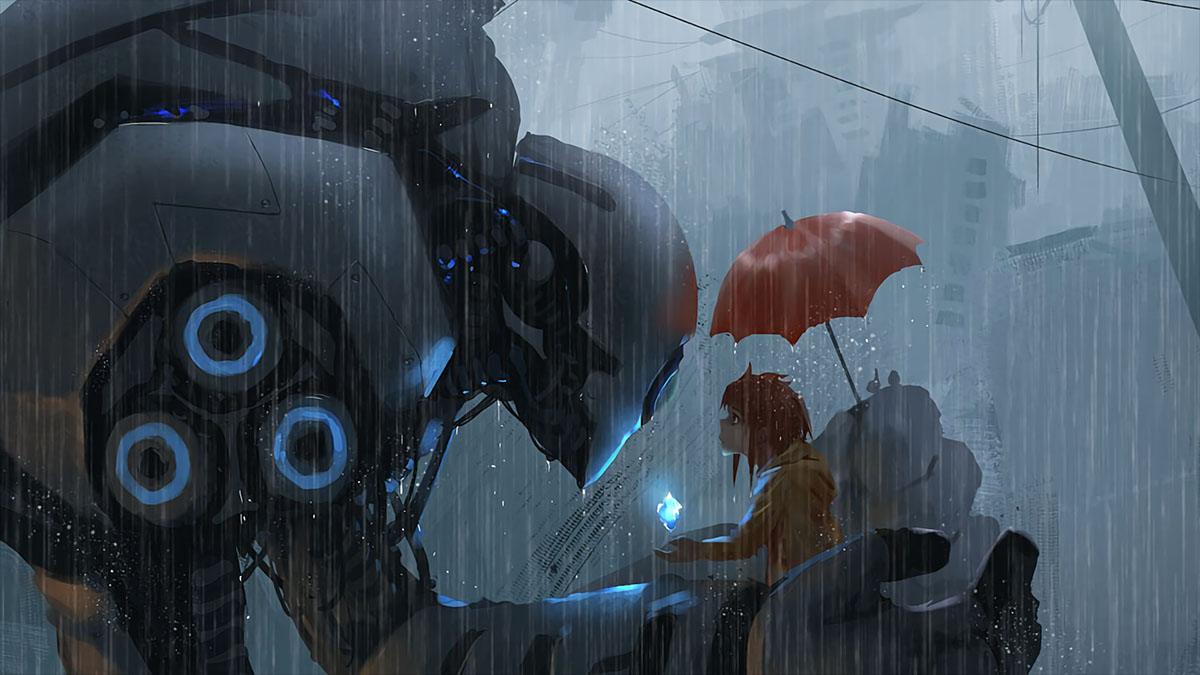
A while back I made a post about the use of robots to simulate evolutionary behaviors by giving them a kind of free reign and copying the programming of the machines most successful in their tasks into the rest of the test group to emulate natural selection. Now, the lead author of that study, Dario Floreano, teamed up with a biologist on a paper recently published in PLoS Biology detailed a whole slew of experiments in which robots replicate the evolutionary algorithms we see in nature with an impressive level of accuracy. Does this mean a robot army is evolving in some cybernetic lab as we speak? Are we about to welcome our machine overlords which are on the verge of evolving intelligence and posing a potential threat to our dominance of Earth? Well, I wouldn’t worry about that yet. Instead, I’d rather take a look at how and why these robots can mimic evolution.
The first question one might ask is how robots would learn how to make their way through a maze and have a capacity for improvement. Instead of obeying a rigid, hardcoded algorithm, they use artificial neural networks which emulate the statistical model of learning for a computer. The robot is built with sensors which can tell it how far away from a wall or an obstacle it is and based on the information it collects, it makes decisions as to how it will move. Each sensor’s input is assigned an importance and as these sensors do a better and better job of keeping it away from walls, their importance is altered to reflect their usefulness. Out of say, six sensors or so, the machine uses primarily three to guide it where it needs to go and the other three are treated as less useful secondary input. Depending on which sensors are assigned the highest importance, the robot moves in a particular way. It can stay close to the walls or move cleanly down the center of the passages. Either way is fine as long as it’s not bumping into walls on a regular basis in awkward zigzagging motions.
Same principles apply in more elaborate behaviors. Decisions that allow it to succeed in vaguely defined set of rules for an experiment adjust the value of the relevant input and output nodes (often referred to as neurons since that’s what they try to simulate), and by keeping the successful networks around, the researchers allow the behavior to morph even more and gain new nuances. The machines are able not to just travel mazes, but work in teams, emulate an evolutionary arms race between predator and prey, find a home base, and there’s even a hint of the ability to change their bodies when given the right technology based on physics simulations in specialized design programs. In short, evolutionary algorithms are very powerful and by giving machines a degree of freedom and randomness, we’re giving them the chance to develop new ways to tackle problems. I wouldn’t recommend this to business application designers who need a predictable way to manage data but for researchers who need to build robots for exotic applications, like exploring alien planets, trying to replicate evolution in metal, plastic, and silicon may be the way to go.
Lastly, we should note that while the robots seem to be doing things we would associate with intelligence, i.e. learning, memory, strategy and some semblance creativity, they’re not self-aware. You could say they’re not a sapient machine but certainly a sentient one. And they do have limits imposed on them by designers. Even if they’re given the ability to change their bodies, they can only do so much with the building blocks they’re given and it’s up to the designer if they’ll get new ones. Likewise, they also can’t handle major changes in the overall structure of their bodies and circuits. They’ll simply break down or malfunction because they’re still machines and the basic rules of electronics and engineering still apply. Until we could recreate swarming robots able to communicate with each other and work on a scale comparable to cells, macro machinery just won’t have the plasticity of living things. Our robots’ dependence on us designing their vital components actually holds them back and it seems like the best design for hyper-advanced robots of the future may be to do as little designing as possible at all to allow the machines to go through millions of permutations on their own.
However, even under with the most hands off designs, our machines won’t be sapient, even though it might take a lot less effort to simulate something like a sense of self-awareness than we may think according to some cutting edge research. Then again, to be as scientific about this as we can, we have to ask whether the consciousness based on repeating cognitive circuits will be anything like the consciousness we’d be able to recognize as similar to our own. After all, we still don’t have a consensus on how to define intelligence, and it seems just as difficult to define consciousness in objective, empirical terms…
See: Floreano, D., et. al., (2010). Evolution of Adaptive Behaviour in Robots by Means of Darwinian Selection PLoS Biology, 8 (1) DOI: 10.1371/journal.pbio.1000292
[ story suggestion by Kate Sherrod ]