the oncology revolution that wasn’t
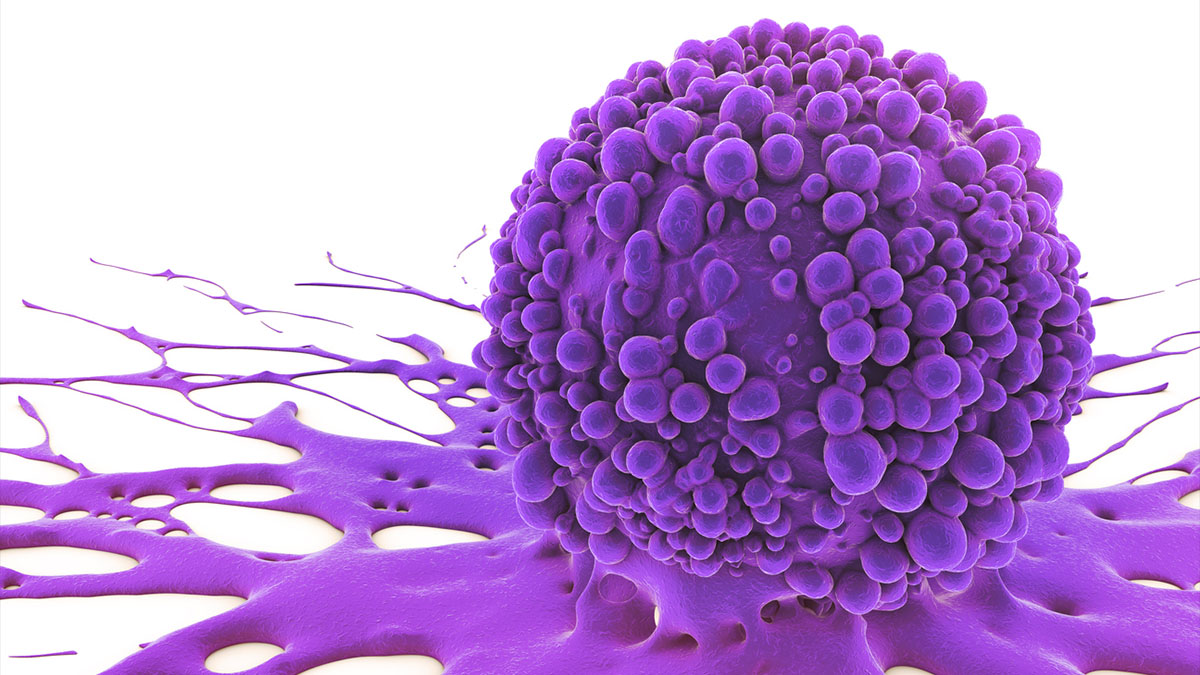
As we’re living longer and longer, some form of cancer is becoming one of the top causes of death. Research indicates that in the UK and Canada, half the population can expect a cancer diagnosis, while in the U.S. it’s one third. Considering that there are over 200 types of cancer and how difficult they can be to treat, the current holy grail of treatment is to reduce the disease to a chronic condition with managed flare-ups. The best way to do that is to know much more about both the patients and the different forms of cancer with which they can be afflicted, and with too much data to sort through by humans alone, doctors were hoping that artificial intelligence could come to the rescue.
Ideally, you would collect as much data as you possibly can about a patient and the specific cancer he or she had, as well as detailed notes about response to treatment and whether the disease went in remission, returned, or metastasized. With trillions of data points, patterns and connections should’ve emerged, allowing doctors to adjust treatment plans and quickly test new approaches for patients who needed creative ideas to stave off death. And that was what IBM sold oncologists in Watson, a machine supposedly capable of doing just that. What it delivered was nothing more than clinical notes from New York’s Memorial Sloan Kettering Cancer Center in what is functionally an interactive flowchart.
When you teach an artificial intelligence, its training set has to include real world data with the inputs you received and outcomes you observed. The learning algorithms will then try to find connections that influenced the outcome by essentially guessing until they get it right. This allows them to “understand” what inputs are most important and the odds of a desired outcome from a new data set. Watson went through a similar process, but only from data provided by the oncologists at Sloan Kettering and heavily biased to replicate their exact approaches and treatment plans. This is why it’s not surprising that a number of hospitals around the world rejected it after finding that methods they saw work were discarded by the AI in test runs.
To an extent, one can see why IBM handed over the training to a respected cancer hospital. Without a baseline for competently recommending decent treatment plans, Watson would have no idea what to recommend and could tell doctors to use leeches or try turning the patient into Deadpool. To make Watson truly innovative would’ve taken many years of collecting petabytes of of data from doctors all over the world and analyzing treatments and outcomes with far more rigor than anyone ever had, requiring both global coordination and very significant investment. But instead of finding a middle ground and releasing an AI with a baseline but open to collecting any and all clinical data, they chose to simply stick to that baseline with slight updates.
And that’s actually a very saddening turn of events. Artificial intelligence is being billed as a sort of black box powered by magic and capable of finding answers to questions you didn’t even know you had. Behind the catchy marketing, however, are well understood algorithms of varying complexity ran at different scales. Implement them poorly or feed them incomplete or extremely biased data, and you end up with crime predicting systems that can’t actually predict crime, race-blind systems that heavily discriminate against minorities, and yes, a cancer research platform that just parrots what one group of researchers says is right.
“Machines can’t help us find cures for cancers” or “AI is all hype” are not the messages doctors should take away from all this, and after dealing with a technology that failed to live up to the hype because its creators ignored the basics of making an artificial intelligence useful it’s hard not to imagine that some will. It would be very unfortunate if this impression isn’t corrected and no one challenges how IBM chose to restrain Watson’s understanding of oncology with a rival project. We desperately need machines capable of crunching through enormous databases of medical data to tease out correlations and novel approaches we missed or ignored, and if Watson isn’t going to do it, the space is wide open to those willing to do AI right.